2022-12-19
来源:华纳网
责任编辑:王双双
人气:
核心提示:本节我们使用Android结合飞桨人工智能开源套件来实现口罩佩戴检测以下是部分代码#include "Pipeline.h"
大家好,欢迎来到谷雨课堂
本节我们使用Android结合飞桨人工智能开源套件
来实现口罩佩戴检测
以下是部分代码
#include "Pipeline.h"
FaceDetector::FaceDetector(const std::string &modelDir, const int cpuThreadNum,
const std::string &cpuPowerMode, float inputScale,
const std::vector<float> &inputMean,
const std::vector<float> &inputStd,
float scoreThreshold)
: inputScale_(inputScale), inputMean_(inputMean), inputStd_(inputStd),
scoreThreshold_(scoreThreshold) {
paddle::lite_api::MobileConfig config;
config.set_model_from_file(modelDir + "/model.nb");
config.set_threads(cpuThreadNum);
config.set_power_mode(ParsePowerMode(cpuPowerMode));
predictor_ =
paddle::lite_api::CreatePaddlePredictor<paddle::lite_api::MobileConfig>(
config);
}
void FaceDetector::Preprocess(const cv::Mat &rgbaImage) {
auto t = GetCurrentTime();
cv::Mat resizedRGBAImage;
cv::resize(rgbaImage, resizedRGBAImage, cv::Size(), inputScale_, inputScale_);
cv::Mat resizedBGRImage;
cv::cvtColor(resizedRGBAImage, resizedBGRImage, cv::COLOR_RGBA2BGR);
resizedBGRImage.convertTo(resizedBGRImage, CV_32FC3, 1.0 / 255.0f);
std::vector<int64_t> inputShape = {1, 3, resizedBGRImage.rows,
resizedBGRImage.cols};
// Prepare input tensor
auto inputTensor = predictor_->GetInput(0);
inputTensor->Resize(inputShape);
auto inputData = inputTensor->mutable_data<float>();
NHWC2NCHW(reinterpret_cast<const float *>(resizedBGRImage.data), inputData,
inputMean_.data(), inputStd_.data(), inputShape[3], inputShape[2]);
}
void FaceDetector::Postprocess(const cv::Mat &rgbaImage,
std::vector<Face> *faces) {
int imageWidth = rgbaImage.cols;
int imageHeight = rgbaImage.rows;
// Get output tensor
auto outputTensor = predictor_->GetOutput(2);
auto outputData = outputTensor->data<float>();
auto outputShape = outputTensor->shape();
int outputSize = ShapeProduction(outputShape);
faces->clear();
for (int i = 0; i < outputSize; i += 6) {
// Class id
float class_id = outputData[i];
// Confidence score
float score = outputData[i + 1];
int left = outputData[i + 2] * imageWidth;
int top = outputData[i + 3] * imageHeight;
int right = outputData[i + 4] * imageWidth;
int bottom = outputData[i + 5] * imageHeight;
int width = right - left;
int height = bottom - top;
if (score > scoreThreshold_) {
Face face;
face.roi = cv::Rect(left, top, width, height) &
cv::Rect(0, 0, imageWidth - 1, imageHeight - 1);
faces->push_back(face);
}
}
}
void FaceDetector::Predict(const cv::Mat &rgbaImage, std::vector<Face> *faces,
double *preprocessTime, double *predictTime,
double *postprocessTime) {
auto t = GetCurrentTime();
t = GetCurrentTime();
Preprocess(rgbaImage);
*preprocessTime = GetElapsedTime(t);
LOGD("Face detector postprocess costs %f ms", *preprocessTime);
t = GetCurrentTime();
predictor_->Run();
*predictTime = GetElapsedTime(t);
LOGD("Face detector predict costs %f ms", *predictTime);
t = GetCurrentTime();
Postprocess(rgbaImage, faces);
*postprocessTime = GetElapsedTime(t);
LOGD("Face detector postprocess costs %f ms", *postprocessTime);
}
MaskClassifier::MaskClassifier(const std::string &modelDir,
const int cpuThreadNum,
const std::string &cpuPowerMode, int inputWidth,
int inputHeight,
const std::vector<float> &inputMean,
const std::vector<float> &inputStd)
: inputWidth_(inputWidth), inputHeight_(inputHeight), inputMean_(inputMean),
inputStd_(inputStd) {
paddle::lite_api::MobileConfig config;
config.set_model_from_file(modelDir + "/model.nb");
config.set_threads(cpuThreadNum);
config.set_power_mode(ParsePowerMode(cpuPowerMode));
predictor_ =
paddle::lite_api::CreatePaddlePredictor<paddle::lite_api::MobileConfig>(
config);
}
void MaskClassifier::Preprocess(const cv::Mat &rgbaImage,
const std::vector<Face> &faces) {
// Prepare input tensor
auto inputTensor = predictor_->GetInput(0);
int batchSize = faces.size();
std::vector<int64_t> inputShape = {batchSize, 3, inputHeight_, inputWidth_};
inputTensor->Resize(inputShape);
auto inputData = inputTensor->mutable_data<float>();
for (int i = 0; i < batchSize; i++) {
// Adjust the face region to improve the accuracy according to the aspect
// ratio of input image of the target model
int cx = faces[i].roi.x + faces[i].roi.width / 2.0f;
int cy = faces[i].roi.y + faces[i].roi.height / 2.0f;
int w = faces[i].roi.width;
int h = faces[i].roi.height;
float roiAspectRatio =
static_cast<float>(faces[i].roi.width) / faces[i].roi.height;
float inputAspectRatio = static_cast<float>(inputShape[3]) / inputShape[2];
if (fabs(roiAspectRatio - inputAspectRatio) > 1e-5) {
float widthRatio = static_cast<float>(faces[i].roi.width) / inputShape[3];
float heightRatio =
static_cast<float>(faces[i].roi.height) / inputShape[2];
if (widthRatio > heightRatio) {
h = w / inputAspectRatio;
} else {
w = h * inputAspectRatio;
}
}
cv::Mat resizedRGBAImage(
rgbaImage, cv::Rect(cx - w / 2, cy - h / 2, w, h) &
cv::Rect(0, 0, rgbaImage.cols - 1, rgbaImage.rows - 1));
cv::resize(resizedRGBAImage, resizedRGBAImage,
cv::Size(inputShape[3], inputShape[2]));
cv::Mat resizedBGRImage;
cv::cvtColor(resizedRGBAImage, resizedBGRImage, cv::COLOR_RGBA2BGR);
resizedBGRImage.convertTo(resizedBGRImage, CV_32FC3, 1.0 / 255.0f);
NHWC2NCHW(reinterpret_cast<const float *>(resizedBGRImage.data), inputData,
inputMean_.data(), inputStd_.data(), inputShape[3],
inputShape[2]);
inputData += inputShape[1] * inputShape[2] * inputShape[3];
}
}
void MaskClassifier::Postprocess(std::vector<Face> *faces) {
auto outputTensor = predictor_->GetOutput(0);
auto outputData = outputTensor->data<float>();
auto outputShape = outputTensor->shape();
int outputSize = ShapeProduction(outputShape);
int batchSize = faces->size();
int classNum = outputSize / batchSize;
for (int i = 0; i < batchSize; i++) {
(*faces)[i].classid = 0;
(*faces)[i].confidence = *(outputData++);
for (int j = 1; j < classNum; j++) {
auto confidence = *(outputData++);
if (confidence > (*faces)[i].confidence) {
(*faces)[i].classid = j;
(*faces)[i].confidence = confidence;
}
}
}
}
void MaskClassifier::Predict(const cv::Mat &rgbaImage, std::vector<Face> *faces,
double *preprocessTime, double *predictTime,
double *postprocessTime) {
auto t = GetCurrentTime();
t = GetCurrentTime();
Preprocess(rgbaImage, *faces);
*preprocessTime = GetElapsedTime(t);
LOGD("Mask classifier postprocess costs %f ms", *preprocessTime);
t = GetCurrentTime();
predictor_->Run();
*predictTime = GetElapsedTime(t);
LOGD("Mask classifier predict costs %f ms", *predictTime);
t = GetCurrentTime();
Postprocess(faces);
*postprocessTime = GetElapsedTime(t);
LOGD("Mask classifier postprocess costs %f ms", *postprocessTime);
}
Pipeline::Pipeline(const std::string &fdtModelDir, const int fdtCPUThreadNum,
const std::string &fdtCPUPowerMode, float fdtInputScale,
const std::vector<float> &fdtInputMean,
const std::vector<float> &fdtInputStd,
float detScoreThreshold, const std::string &mclModelDir,
const int mclCPUThreadNum,
const std::string &mclCPUPowerMode, int mclInputWidth,
int mclInputHeight, const std::vector<float> &mclInputMean,
const std::vector<float> &mclInputStd) {
faceDetector_.reset(new FaceDetector(
fdtModelDir, fdtCPUThreadNum, fdtCPUPowerMode, fdtInputScale,
fdtInputMean, fdtInputStd, detScoreThreshold));
maskClassifier_.reset(new MaskClassifier(
mclModelDir, mclCPUThreadNum, mclCPUPowerMode, mclInputWidth,
mclInputHeight, mclInputMean, mclInputStd));
}
void Pipeline::VisualizeResults(const std::vector<Face> &faces,
cv::Mat *rgbaImage) {
for (int i = 0; i < faces.size(); i++) {
auto roi = faces[i].roi;
// Configure color and text size
cv::Scalar color;
std::string text;
if (faces[i].classid == 1) {
text = "MASK: ";
color = cv::Scalar(0, 255, 0);
} else {
text = "NO MASK: ";
color = cv::Scalar(255, 0, 0);
}
text += std::to_string(static_cast<int>(faces[i].confidence * 100)) + "%";
int font_face = cv::FONT_HERSHEY_PLAIN;
double font_scale = 1.f;
float thickness = 1;
cv::Size text_size =
cv::getTextSize(text, font_face, font_scale, thickness, nullptr);
font_scale = faces[i].roi.width * font_scale / text_size.width;
text_size =
cv::getTextSize(text, font_face, font_scale, thickness, nullptr);
// Draw roi object, text and background
cv::rectangle(*rgbaImage, faces[i].roi, color, 2);
cv::rectangle(
*rgbaImage,
cv::Point2d(faces[i].roi.x,
faces[i].roi.y - round(text_size.height * 1.25f)),
cv::Point2d(faces[i].roi.x + faces[i].roi.width, faces[i].roi.y), color,
-1);
cv::putText(*rgbaImage, text, cv::Point2d(faces[i].roi.x, faces[i].roi.y),
font_face, font_scale, cv::Scalar(255, 255, 255), thickness);
}
}
void Pipeline::VisualizeStatus(double readGLFBOTime, double writeGLTextureTime,
double fdtPreprocessTime, double fdtPredictTime,
double fdtPostprocessTime,
double mclPreprocessTime, double mclPredictTime,
double mclPostprocessTime, cv::Mat *rgbaImage) {
char text[255];
cv::Scalar color = cv::Scalar(255, 255, 255);
int font_face = cv::FONT_HERSHEY_PLAIN;
double font_scale = 1.f;
float thickness = 1;
sprintf(text, "Read GLFBO time: %.1f ms", readGLFBOTime);
cv::Size text_size =
cv::getTextSize(text, font_face, font_scale, thickness, nullptr);
text_size.height *= 1.25f;
cv::Point2d offset(10, text_size.height + 15);
cv::putText(*rgbaImage, text, offset, font_face, font_scale, color,
thickness);
sprintf(text, "Write GLTexture time: %.1f ms", writeGLTextureTime);
offset.y += text_size.height;
cv::putText(*rgbaImage, text, offset, font_face, font_scale, color,
thickness);
// Face detector
sprintf(text, "FDT preprocess time: %.1f ms", fdtPreprocessTime);
offset.y += text_size.height;
cv::putText(*rgbaImage, text, offset, font_face, font_scale, color,
thickness);
sprintf(text, "FDT predict time: %.1f ms", fdtPredictTime);
offset.y += text_size.height;
cv::putText(*rgbaImage, text, offset, font_face, font_scale, color,
thickness);
sprintf(text, "FDT postprocess time: %.1f ms", fdtPostprocessTime);
offset.y += text_size.height;
cv::putText(*rgbaImage, text, offset, font_face, font_scale, color,
thickness);
// Mask classification
sprintf(text, "MCL preprocess time: %.1f ms", mclPreprocessTime);
offset.y += text_size.height;
cv::putText(*rgbaImage, text, offset, font_face, font_scale, color,
thickness);
sprintf(text, "MCL predict time: %.1f ms", mclPredictTime);
offset.y += text_size.height;
cv::putText(*rgbaImage, text, offset, font_face, font_scale, color,
thickness);
sprintf(text, "MCL postprocess time: %.1f ms", mclPostprocessTime);
offset.y += text_size.height;
cv::putText(*rgbaImage, text, offset, font_face, font_scale, color,
thickness);
}
bool Pipeline::Process(int inTexureId, int outTextureId, int textureWidth,
int textureHeight, std::string savedImagePath) {
double readGLFBOTime = 0, writeGLTextureTime = 0;
double fdtPreprocessTime = 0, fdtPredictTime = 0, fdtPostprocessTime = 0;
double mclPreprocessTime = 0, mclPredictTime = 0, mclPostprocessTime = 0;
cv::Mat rgbaImage;
CreateRGBAImageFromGLFBOTexture(textureWidth, textureHeight, &rgbaImage,
&readGLFBOTime);
// Stage1: Face detection
std::vector<Face> faces;
faceDetector_->Predict(rgbaImage, &faces, &fdtPreprocessTime, &fdtPredictTime,
&fdtPostprocessTime);
if (faces.size() > 0) {
// Stage2: Mask wearing classification
maskClassifier_->Predict(rgbaImage, &faces, &mclPreprocessTime,
&mclPredictTime, &mclPostprocessTime);
// Stage3: Visualize results
VisualizeResults(faces, &rgbaImage);
}
// Visualize the status(performace data) to origin image
VisualizeStatus(readGLFBOTime, writeGLTextureTime, fdtPreprocessTime,
fdtPredictTime, fdtPostprocessTime, mclPreprocessTime,
mclPredictTime, mclPostprocessTime, &rgbaImage);
// Dump modified image if savedImagePath is set
if (!savedImagePath.empty()) {
cv::Mat bgrImage;
cv::cvtColor(rgbaImage, bgrImage, cv::COLOR_RGBA2BGR);
imwrite(savedImagePath, bgrImage);
}
WriteRGBAImageBackToGLTexture(rgbaImage, outTextureId, &writeGLTextureTime);
return true;
}
package com.baidu.paddle.lite.demo.mask_detection;
import android.Manifest;
import android.app.Activity;
import android.app.AlertDialog;
import android.content.DialogInterface;
import android.content.Intent;
import android.content.SharedPreferences;
import android.content.pm.PackageManager;
import android.graphics.*;
import android.os.Bundle;
import android.preference.PreferenceManager;
import android.support.annotation.NonNull;
import android.support.v4.app.ActivityCompat;
import android.support.v4.content.ContextCompat;
import android.util.Log;
import android.view.*;
import android.widget.*;
import com.baidu.paddle.lite.demo.common.CameraSurfaceView;
import com.baidu.paddle.lite.demo.common.Utils;
import java.io.File;
import java.nio.file.attribute.FileTime;
import java.text.SimpleDateFormat;
import java.util.Date;
public class MainActivity extends Activity implements View.OnClickListener, CameraSurfaceView.OnTextureChangedListener {
private static final String TAG = MainActivity.class.getSimpleName();
CameraSurfaceView svPreview;
TextView tvStatus;
ImageButton btnSwitch;
ImageButton btnShutter;
ImageButton btnSettings;
String savedImagePath = "";
int lastFrameIndex = 0;
long lastFrameTime;
Native predictor = new Native();
@Override
protected void onCreate(Bundle savedInstanceState) {
super.onCreate(savedInstanceState);
// Fullscreen
requestWindowFeature(Window.FEATURE_NO_TITLE);
getWindow().setFlags(WindowManager.LayoutParams.FLAG_FULLSCREEN, WindowManager.LayoutParams.FLAG_FULLSCREEN);
setContentView(R.layout.activity_main);
// Clear all setting items to avoid app crashing due to the incorrect settings
initSettings();
// Init the camera preview and UI components
initView();
// Check and request CAMERA and WRITE_EXTERNAL_STORAGE permissions
if (!checkAllPermissions()) {
requestAllPermissions();
}
}
@Override
public void onClick(View v) {
switch (v.getId()) {
case R.id.btn_switch:
svPreview.switchCamera();
break;
case R.id.btn_shutter:
SimpleDateFormat date = new SimpleDateFormat("yyyy_MM_dd_HH_mm_ss");
synchronized (this) {
savedImagePath = Utils.getDCIMDirectory() + File.separator + date.format(new Date()).toString() + ".png";
}
Toast.makeText(MainActivity.this, "Save snapshot to " + savedImagePath, Toast.LENGTH_SHORT).show();
break;
case R.id.btn_settings:
startActivity(new Intent(MainActivity.this, SettingsActivity.class));
break;
}
}
@Override
public boolean onTextureChanged(int inTextureId, int outTextureId, int textureWidth, int textureHeight) {
String savedImagePath = "";
synchronized (this) {
savedImagePath = MainActivity.this.savedImagePath;
}
boolean modified = predictor.process(inTextureId, outTextureId, textureWidth, textureHeight, savedImagePath);
if (!savedImagePath.isEmpty()) {
synchronized (this) {
MainActivity.this.savedImagePath = "";
}
}
lastFrameIndex++;
if (lastFrameIndex >= 30) {
final int fps = (int) (lastFrameIndex * 1e9 / (System.nanoTime() - lastFrameTime));
runOnUiThread(new Runnable() {
public void run() {
tvStatus.setText(Integer.toString(fps) + "fps");
}
});
lastFrameIndex = 0;
lastFrameTime = System.nanoTime();
}
return modified;
}
@Override
protected void onResume() {
super.onResume();
// Reload settings and re-initialize the predictor
checkAndUpdateSettings();
// Open camera until the permissions have been granted
if (!checkAllPermissions()) {
svPreview.disableCamera();
}
svPreview.onResume();
}
@Override
protected void onPause() {
super.onPause();
svPreview.onPause();
}
@Override
protected void onDestroy() {
if (predictor != null) {
predictor.release();
}
super.onDestroy();
}
public void initView() {
svPreview = (CameraSurfaceView) findViewById(R.id.sv_preview);
svPreview.setOnTextureChangedListener(this);
tvStatus = (TextView) findViewById(R.id.tv_status);
btnSwitch = (ImageButton) findViewById(R.id.btn_switch);
btnSwitch.setOnClickListener(this);
btnShutter = (ImageButton) findViewById(R.id.btn_shutter);
btnShutter.setOnClickListener(this);
btnSettings = (ImageButton) findViewById(R.id.btn_settings);
btnSettings.setOnClickListener(this);
}
public void initSettings() {
SharedPreferences sharedPreferences = PreferenceManager.getDefaultSharedPreferences(this);
SharedPreferences.Editor editor = sharedPreferences.edit();
editor.clear();
editor.commit();
}
public void checkAndUpdateSettings() {
if (SettingsActivity.checkAndUpdateSettings(this)) {
String fdtRealModelDir = getCacheDir() + "/" + SettingsActivity.fdtModelDir;
Utils.copyDirectoryFromAssets(this, SettingsActivity.fdtModelDir, fdtRealModelDir);
String mclRealModelDir = getCacheDir() + "/" + SettingsActivity.mclModelDir;
Utils.copyDirectoryFromAssets(this, SettingsActivity.mclModelDir, mclRealModelDir);
predictor.init(
fdtRealModelDir,
SettingsActivity.fdtCPUThreadNum,
SettingsActivity.fdtCPUPowerMode,
SettingsActivity.fdtInputScale,
SettingsActivity.fdtInputMean,
SettingsActivity.fdtInputStd,
SettingsActivity.fdtScoreThreshold,
mclRealModelDir,
SettingsActivity.mclCPUThreadNum,
SettingsActivity.mclCPUPowerMode,
SettingsActivity.mclInputWidth,
SettingsActivity.mclInputHeight,
SettingsActivity.mclInputMean,
SettingsActivity.mclInputStd);
}
}
@Override
public void onRequestPermissionsResult(int requestCode, @NonNull String[] permissions,
@NonNull int[] grantResults) {
super.onRequestPermissionsResult(requestCode, permissions, grantResults);
if (grantResults[0] != PackageManager.PERMISSION_GRANTED || grantResults[1] != PackageManager.PERMISSION_GRANTED) {
new AlertDialog.Builder(MainActivity.this)
.setTitle("Permission denied")
.setMessage("Click to force quit the app, then open Settings->Apps & notifications->Target " +
"App->Permissions to grant all of the permissions.")
.setCancelable(false)
.setPositiveButton("Exit", new DialogInterface.OnClickListener() {
@Override
public void onClick(DialogInterface dialog, int which) {
MainActivity.this.finish();
}
}).show();
}
}
private void requestAllPermissions() {
ActivityCompat.requestPermissions(this, new String[]{Manifest.permission.WRITE_EXTERNAL_STORAGE,
Manifest.permission.CAMERA}, 0);
}
private boolean checkAllPermissions() {
return ContextCompat.checkSelfPermission(this, Manifest.permission.WRITE_EXTERNAL_STORAGE) == PackageManager.PERMISSION_GRANTED
&& ContextCompat.checkSelfPermission(this, Manifest.permission.CAMERA) == PackageManager.PERMISSION_GRANTED;
}
}
完整的源代码可以登录【华纳网】下载。
https://www.worldwarner.com/
免责声明:本文仅代表作者个人观点,与华纳网无关。其原创性以及文中陈述文字和内容未经本站证实,对本文以及其中全部或者部分内容、文字的真实性、完整性、及时性本站不作任何保证或承诺,请读者仅作参考,并请自行核实相关内容。
本节我们使用Android结合飞桨人工智能开源套件
来实现口罩佩戴检测
以下是部分代码
#include "Pipeline.h"
FaceDetector::FaceDetector(const std::string &modelDir, const int cpuThreadNum,
const std::string &cpuPowerMode, float inputScale,
const std::vector<float> &inputMean,
const std::vector<float> &inputStd,
float scoreThreshold)
: inputScale_(inputScale), inputMean_(inputMean), inputStd_(inputStd),
scoreThreshold_(scoreThreshold) {
paddle::lite_api::MobileConfig config;
config.set_model_from_file(modelDir + "/model.nb");
config.set_threads(cpuThreadNum);
config.set_power_mode(ParsePowerMode(cpuPowerMode));
predictor_ =
paddle::lite_api::CreatePaddlePredictor<paddle::lite_api::MobileConfig>(
config);
}
void FaceDetector::Preprocess(const cv::Mat &rgbaImage) {
auto t = GetCurrentTime();
cv::Mat resizedRGBAImage;
cv::resize(rgbaImage, resizedRGBAImage, cv::Size(), inputScale_, inputScale_);
cv::Mat resizedBGRImage;
cv::cvtColor(resizedRGBAImage, resizedBGRImage, cv::COLOR_RGBA2BGR);
resizedBGRImage.convertTo(resizedBGRImage, CV_32FC3, 1.0 / 255.0f);
std::vector<int64_t> inputShape = {1, 3, resizedBGRImage.rows,
resizedBGRImage.cols};
// Prepare input tensor
auto inputTensor = predictor_->GetInput(0);
inputTensor->Resize(inputShape);
auto inputData = inputTensor->mutable_data<float>();
NHWC2NCHW(reinterpret_cast<const float *>(resizedBGRImage.data), inputData,
inputMean_.data(), inputStd_.data(), inputShape[3], inputShape[2]);
}
void FaceDetector::Postprocess(const cv::Mat &rgbaImage,
std::vector<Face> *faces) {
int imageWidth = rgbaImage.cols;
int imageHeight = rgbaImage.rows;
// Get output tensor
auto outputTensor = predictor_->GetOutput(2);
auto outputData = outputTensor->data<float>();
auto outputShape = outputTensor->shape();
int outputSize = ShapeProduction(outputShape);
faces->clear();
for (int i = 0; i < outputSize; i += 6) {
// Class id
float class_id = outputData[i];
// Confidence score
float score = outputData[i + 1];
int left = outputData[i + 2] * imageWidth;
int top = outputData[i + 3] * imageHeight;
int right = outputData[i + 4] * imageWidth;
int bottom = outputData[i + 5] * imageHeight;
int width = right - left;
int height = bottom - top;
if (score > scoreThreshold_) {
Face face;
face.roi = cv::Rect(left, top, width, height) &
cv::Rect(0, 0, imageWidth - 1, imageHeight - 1);
faces->push_back(face);
}
}
}
void FaceDetector::Predict(const cv::Mat &rgbaImage, std::vector<Face> *faces,
double *preprocessTime, double *predictTime,
double *postprocessTime) {
auto t = GetCurrentTime();
t = GetCurrentTime();
Preprocess(rgbaImage);
*preprocessTime = GetElapsedTime(t);
LOGD("Face detector postprocess costs %f ms", *preprocessTime);
t = GetCurrentTime();
predictor_->Run();
*predictTime = GetElapsedTime(t);
LOGD("Face detector predict costs %f ms", *predictTime);
t = GetCurrentTime();
Postprocess(rgbaImage, faces);
*postprocessTime = GetElapsedTime(t);
LOGD("Face detector postprocess costs %f ms", *postprocessTime);
}
MaskClassifier::MaskClassifier(const std::string &modelDir,
const int cpuThreadNum,
const std::string &cpuPowerMode, int inputWidth,
int inputHeight,
const std::vector<float> &inputMean,
const std::vector<float> &inputStd)
: inputWidth_(inputWidth), inputHeight_(inputHeight), inputMean_(inputMean),
inputStd_(inputStd) {
paddle::lite_api::MobileConfig config;
config.set_model_from_file(modelDir + "/model.nb");
config.set_threads(cpuThreadNum);
config.set_power_mode(ParsePowerMode(cpuPowerMode));
predictor_ =
paddle::lite_api::CreatePaddlePredictor<paddle::lite_api::MobileConfig>(
config);
}
void MaskClassifier::Preprocess(const cv::Mat &rgbaImage,
const std::vector<Face> &faces) {
// Prepare input tensor
auto inputTensor = predictor_->GetInput(0);
int batchSize = faces.size();
std::vector<int64_t> inputShape = {batchSize, 3, inputHeight_, inputWidth_};
inputTensor->Resize(inputShape);
auto inputData = inputTensor->mutable_data<float>();
for (int i = 0; i < batchSize; i++) {
// Adjust the face region to improve the accuracy according to the aspect
// ratio of input image of the target model
int cx = faces[i].roi.x + faces[i].roi.width / 2.0f;
int cy = faces[i].roi.y + faces[i].roi.height / 2.0f;
int w = faces[i].roi.width;
int h = faces[i].roi.height;
float roiAspectRatio =
static_cast<float>(faces[i].roi.width) / faces[i].roi.height;
float inputAspectRatio = static_cast<float>(inputShape[3]) / inputShape[2];
if (fabs(roiAspectRatio - inputAspectRatio) > 1e-5) {
float widthRatio = static_cast<float>(faces[i].roi.width) / inputShape[3];
float heightRatio =
static_cast<float>(faces[i].roi.height) / inputShape[2];
if (widthRatio > heightRatio) {
h = w / inputAspectRatio;
} else {
w = h * inputAspectRatio;
}
}
cv::Mat resizedRGBAImage(
rgbaImage, cv::Rect(cx - w / 2, cy - h / 2, w, h) &
cv::Rect(0, 0, rgbaImage.cols - 1, rgbaImage.rows - 1));
cv::resize(resizedRGBAImage, resizedRGBAImage,
cv::Size(inputShape[3], inputShape[2]));
cv::Mat resizedBGRImage;
cv::cvtColor(resizedRGBAImage, resizedBGRImage, cv::COLOR_RGBA2BGR);
resizedBGRImage.convertTo(resizedBGRImage, CV_32FC3, 1.0 / 255.0f);
NHWC2NCHW(reinterpret_cast<const float *>(resizedBGRImage.data), inputData,
inputMean_.data(), inputStd_.data(), inputShape[3],
inputShape[2]);
inputData += inputShape[1] * inputShape[2] * inputShape[3];
}
}
void MaskClassifier::Postprocess(std::vector<Face> *faces) {
auto outputTensor = predictor_->GetOutput(0);
auto outputData = outputTensor->data<float>();
auto outputShape = outputTensor->shape();
int outputSize = ShapeProduction(outputShape);
int batchSize = faces->size();
int classNum = outputSize / batchSize;
for (int i = 0; i < batchSize; i++) {
(*faces)[i].classid = 0;
(*faces)[i].confidence = *(outputData++);
for (int j = 1; j < classNum; j++) {
auto confidence = *(outputData++);
if (confidence > (*faces)[i].confidence) {
(*faces)[i].classid = j;
(*faces)[i].confidence = confidence;
}
}
}
}
void MaskClassifier::Predict(const cv::Mat &rgbaImage, std::vector<Face> *faces,
double *preprocessTime, double *predictTime,
double *postprocessTime) {
auto t = GetCurrentTime();
t = GetCurrentTime();
Preprocess(rgbaImage, *faces);
*preprocessTime = GetElapsedTime(t);
LOGD("Mask classifier postprocess costs %f ms", *preprocessTime);
t = GetCurrentTime();
predictor_->Run();
*predictTime = GetElapsedTime(t);
LOGD("Mask classifier predict costs %f ms", *predictTime);
t = GetCurrentTime();
Postprocess(faces);
*postprocessTime = GetElapsedTime(t);
LOGD("Mask classifier postprocess costs %f ms", *postprocessTime);
}
Pipeline::Pipeline(const std::string &fdtModelDir, const int fdtCPUThreadNum,
const std::string &fdtCPUPowerMode, float fdtInputScale,
const std::vector<float> &fdtInputMean,
const std::vector<float> &fdtInputStd,
float detScoreThreshold, const std::string &mclModelDir,
const int mclCPUThreadNum,
const std::string &mclCPUPowerMode, int mclInputWidth,
int mclInputHeight, const std::vector<float> &mclInputMean,
const std::vector<float> &mclInputStd) {
faceDetector_.reset(new FaceDetector(
fdtModelDir, fdtCPUThreadNum, fdtCPUPowerMode, fdtInputScale,
fdtInputMean, fdtInputStd, detScoreThreshold));
maskClassifier_.reset(new MaskClassifier(
mclModelDir, mclCPUThreadNum, mclCPUPowerMode, mclInputWidth,
mclInputHeight, mclInputMean, mclInputStd));
}
void Pipeline::VisualizeResults(const std::vector<Face> &faces,
cv::Mat *rgbaImage) {
for (int i = 0; i < faces.size(); i++) {
auto roi = faces[i].roi;
// Configure color and text size
cv::Scalar color;
std::string text;
if (faces[i].classid == 1) {
text = "MASK: ";
color = cv::Scalar(0, 255, 0);
} else {
text = "NO MASK: ";
color = cv::Scalar(255, 0, 0);
}
text += std::to_string(static_cast<int>(faces[i].confidence * 100)) + "%";
int font_face = cv::FONT_HERSHEY_PLAIN;
double font_scale = 1.f;
float thickness = 1;
cv::Size text_size =
cv::getTextSize(text, font_face, font_scale, thickness, nullptr);
font_scale = faces[i].roi.width * font_scale / text_size.width;
text_size =
cv::getTextSize(text, font_face, font_scale, thickness, nullptr);
// Draw roi object, text and background
cv::rectangle(*rgbaImage, faces[i].roi, color, 2);
cv::rectangle(
*rgbaImage,
cv::Point2d(faces[i].roi.x,
faces[i].roi.y - round(text_size.height * 1.25f)),
cv::Point2d(faces[i].roi.x + faces[i].roi.width, faces[i].roi.y), color,
-1);
cv::putText(*rgbaImage, text, cv::Point2d(faces[i].roi.x, faces[i].roi.y),
font_face, font_scale, cv::Scalar(255, 255, 255), thickness);
}
}
void Pipeline::VisualizeStatus(double readGLFBOTime, double writeGLTextureTime,
double fdtPreprocessTime, double fdtPredictTime,
double fdtPostprocessTime,
double mclPreprocessTime, double mclPredictTime,
double mclPostprocessTime, cv::Mat *rgbaImage) {
char text[255];
cv::Scalar color = cv::Scalar(255, 255, 255);
int font_face = cv::FONT_HERSHEY_PLAIN;
double font_scale = 1.f;
float thickness = 1;
sprintf(text, "Read GLFBO time: %.1f ms", readGLFBOTime);
cv::Size text_size =
cv::getTextSize(text, font_face, font_scale, thickness, nullptr);
text_size.height *= 1.25f;
cv::Point2d offset(10, text_size.height + 15);
cv::putText(*rgbaImage, text, offset, font_face, font_scale, color,
thickness);
sprintf(text, "Write GLTexture time: %.1f ms", writeGLTextureTime);
offset.y += text_size.height;
cv::putText(*rgbaImage, text, offset, font_face, font_scale, color,
thickness);
// Face detector
sprintf(text, "FDT preprocess time: %.1f ms", fdtPreprocessTime);
offset.y += text_size.height;
cv::putText(*rgbaImage, text, offset, font_face, font_scale, color,
thickness);
sprintf(text, "FDT predict time: %.1f ms", fdtPredictTime);
offset.y += text_size.height;
cv::putText(*rgbaImage, text, offset, font_face, font_scale, color,
thickness);
sprintf(text, "FDT postprocess time: %.1f ms", fdtPostprocessTime);
offset.y += text_size.height;
cv::putText(*rgbaImage, text, offset, font_face, font_scale, color,
thickness);
// Mask classification
sprintf(text, "MCL preprocess time: %.1f ms", mclPreprocessTime);
offset.y += text_size.height;
cv::putText(*rgbaImage, text, offset, font_face, font_scale, color,
thickness);
sprintf(text, "MCL predict time: %.1f ms", mclPredictTime);
offset.y += text_size.height;
cv::putText(*rgbaImage, text, offset, font_face, font_scale, color,
thickness);
sprintf(text, "MCL postprocess time: %.1f ms", mclPostprocessTime);
offset.y += text_size.height;
cv::putText(*rgbaImage, text, offset, font_face, font_scale, color,
thickness);
}
bool Pipeline::Process(int inTexureId, int outTextureId, int textureWidth,
int textureHeight, std::string savedImagePath) {
double readGLFBOTime = 0, writeGLTextureTime = 0;
double fdtPreprocessTime = 0, fdtPredictTime = 0, fdtPostprocessTime = 0;
double mclPreprocessTime = 0, mclPredictTime = 0, mclPostprocessTime = 0;
cv::Mat rgbaImage;
CreateRGBAImageFromGLFBOTexture(textureWidth, textureHeight, &rgbaImage,
&readGLFBOTime);
// Stage1: Face detection
std::vector<Face> faces;
faceDetector_->Predict(rgbaImage, &faces, &fdtPreprocessTime, &fdtPredictTime,
&fdtPostprocessTime);
if (faces.size() > 0) {
// Stage2: Mask wearing classification
maskClassifier_->Predict(rgbaImage, &faces, &mclPreprocessTime,
&mclPredictTime, &mclPostprocessTime);
// Stage3: Visualize results
VisualizeResults(faces, &rgbaImage);
}
// Visualize the status(performace data) to origin image
VisualizeStatus(readGLFBOTime, writeGLTextureTime, fdtPreprocessTime,
fdtPredictTime, fdtPostprocessTime, mclPreprocessTime,
mclPredictTime, mclPostprocessTime, &rgbaImage);
// Dump modified image if savedImagePath is set
if (!savedImagePath.empty()) {
cv::Mat bgrImage;
cv::cvtColor(rgbaImage, bgrImage, cv::COLOR_RGBA2BGR);
imwrite(savedImagePath, bgrImage);
}
WriteRGBAImageBackToGLTexture(rgbaImage, outTextureId, &writeGLTextureTime);
return true;
}
package com.baidu.paddle.lite.demo.mask_detection;
import android.Manifest;
import android.app.Activity;
import android.app.AlertDialog;
import android.content.DialogInterface;
import android.content.Intent;
import android.content.SharedPreferences;
import android.content.pm.PackageManager;
import android.graphics.*;
import android.os.Bundle;
import android.preference.PreferenceManager;
import android.support.annotation.NonNull;
import android.support.v4.app.ActivityCompat;
import android.support.v4.content.ContextCompat;
import android.util.Log;
import android.view.*;
import android.widget.*;
import com.baidu.paddle.lite.demo.common.CameraSurfaceView;
import com.baidu.paddle.lite.demo.common.Utils;
import java.io.File;
import java.nio.file.attribute.FileTime;
import java.text.SimpleDateFormat;
import java.util.Date;
public class MainActivity extends Activity implements View.OnClickListener, CameraSurfaceView.OnTextureChangedListener {
private static final String TAG = MainActivity.class.getSimpleName();
CameraSurfaceView svPreview;
TextView tvStatus;
ImageButton btnSwitch;
ImageButton btnShutter;
ImageButton btnSettings;
String savedImagePath = "";
int lastFrameIndex = 0;
long lastFrameTime;
Native predictor = new Native();
@Override
protected void onCreate(Bundle savedInstanceState) {
super.onCreate(savedInstanceState);
// Fullscreen
requestWindowFeature(Window.FEATURE_NO_TITLE);
getWindow().setFlags(WindowManager.LayoutParams.FLAG_FULLSCREEN, WindowManager.LayoutParams.FLAG_FULLSCREEN);
setContentView(R.layout.activity_main);
// Clear all setting items to avoid app crashing due to the incorrect settings
initSettings();
// Init the camera preview and UI components
initView();
// Check and request CAMERA and WRITE_EXTERNAL_STORAGE permissions
if (!checkAllPermissions()) {
requestAllPermissions();
}
}
@Override
public void onClick(View v) {
switch (v.getId()) {
case R.id.btn_switch:
svPreview.switchCamera();
break;
case R.id.btn_shutter:
SimpleDateFormat date = new SimpleDateFormat("yyyy_MM_dd_HH_mm_ss");
synchronized (this) {
savedImagePath = Utils.getDCIMDirectory() + File.separator + date.format(new Date()).toString() + ".png";
}
Toast.makeText(MainActivity.this, "Save snapshot to " + savedImagePath, Toast.LENGTH_SHORT).show();
break;
case R.id.btn_settings:
startActivity(new Intent(MainActivity.this, SettingsActivity.class));
break;
}
}
@Override
public boolean onTextureChanged(int inTextureId, int outTextureId, int textureWidth, int textureHeight) {
String savedImagePath = "";
synchronized (this) {
savedImagePath = MainActivity.this.savedImagePath;
}
boolean modified = predictor.process(inTextureId, outTextureId, textureWidth, textureHeight, savedImagePath);
if (!savedImagePath.isEmpty()) {
synchronized (this) {
MainActivity.this.savedImagePath = "";
}
}
lastFrameIndex++;
if (lastFrameIndex >= 30) {
final int fps = (int) (lastFrameIndex * 1e9 / (System.nanoTime() - lastFrameTime));
runOnUiThread(new Runnable() {
public void run() {
tvStatus.setText(Integer.toString(fps) + "fps");
}
});
lastFrameIndex = 0;
lastFrameTime = System.nanoTime();
}
return modified;
}
@Override
protected void onResume() {
super.onResume();
// Reload settings and re-initialize the predictor
checkAndUpdateSettings();
// Open camera until the permissions have been granted
if (!checkAllPermissions()) {
svPreview.disableCamera();
}
svPreview.onResume();
}
@Override
protected void onPause() {
super.onPause();
svPreview.onPause();
}
@Override
protected void onDestroy() {
if (predictor != null) {
predictor.release();
}
super.onDestroy();
}
public void initView() {
svPreview = (CameraSurfaceView) findViewById(R.id.sv_preview);
svPreview.setOnTextureChangedListener(this);
tvStatus = (TextView) findViewById(R.id.tv_status);
btnSwitch = (ImageButton) findViewById(R.id.btn_switch);
btnSwitch.setOnClickListener(this);
btnShutter = (ImageButton) findViewById(R.id.btn_shutter);
btnShutter.setOnClickListener(this);
btnSettings = (ImageButton) findViewById(R.id.btn_settings);
btnSettings.setOnClickListener(this);
}
public void initSettings() {
SharedPreferences sharedPreferences = PreferenceManager.getDefaultSharedPreferences(this);
SharedPreferences.Editor editor = sharedPreferences.edit();
editor.clear();
editor.commit();
}
public void checkAndUpdateSettings() {
if (SettingsActivity.checkAndUpdateSettings(this)) {
String fdtRealModelDir = getCacheDir() + "/" + SettingsActivity.fdtModelDir;
Utils.copyDirectoryFromAssets(this, SettingsActivity.fdtModelDir, fdtRealModelDir);
String mclRealModelDir = getCacheDir() + "/" + SettingsActivity.mclModelDir;
Utils.copyDirectoryFromAssets(this, SettingsActivity.mclModelDir, mclRealModelDir);
predictor.init(
fdtRealModelDir,
SettingsActivity.fdtCPUThreadNum,
SettingsActivity.fdtCPUPowerMode,
SettingsActivity.fdtInputScale,
SettingsActivity.fdtInputMean,
SettingsActivity.fdtInputStd,
SettingsActivity.fdtScoreThreshold,
mclRealModelDir,
SettingsActivity.mclCPUThreadNum,
SettingsActivity.mclCPUPowerMode,
SettingsActivity.mclInputWidth,
SettingsActivity.mclInputHeight,
SettingsActivity.mclInputMean,
SettingsActivity.mclInputStd);
}
}
@Override
public void onRequestPermissionsResult(int requestCode, @NonNull String[] permissions,
@NonNull int[] grantResults) {
super.onRequestPermissionsResult(requestCode, permissions, grantResults);
if (grantResults[0] != PackageManager.PERMISSION_GRANTED || grantResults[1] != PackageManager.PERMISSION_GRANTED) {
new AlertDialog.Builder(MainActivity.this)
.setTitle("Permission denied")
.setMessage("Click to force quit the app, then open Settings->Apps & notifications->Target " +
"App->Permissions to grant all of the permissions.")
.setCancelable(false)
.setPositiveButton("Exit", new DialogInterface.OnClickListener() {
@Override
public void onClick(DialogInterface dialog, int which) {
MainActivity.this.finish();
}
}).show();
}
}
private void requestAllPermissions() {
ActivityCompat.requestPermissions(this, new String[]{Manifest.permission.WRITE_EXTERNAL_STORAGE,
Manifest.permission.CAMERA}, 0);
}
private boolean checkAllPermissions() {
return ContextCompat.checkSelfPermission(this, Manifest.permission.WRITE_EXTERNAL_STORAGE) == PackageManager.PERMISSION_GRANTED
&& ContextCompat.checkSelfPermission(this, Manifest.permission.CAMERA) == PackageManager.PERMISSION_GRANTED;
}
}
完整的源代码可以登录【华纳网】下载。
https://www.worldwarner.com/
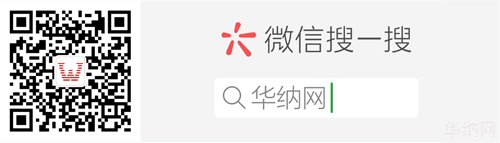
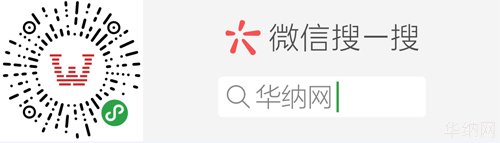
免责声明:本文仅代表作者个人观点,与华纳网无关。其原创性以及文中陈述文字和内容未经本站证实,对本文以及其中全部或者部分内容、文字的真实性、完整性、及时性本站不作任何保证或承诺,请读者仅作参考,并请自行核实相关内容。